
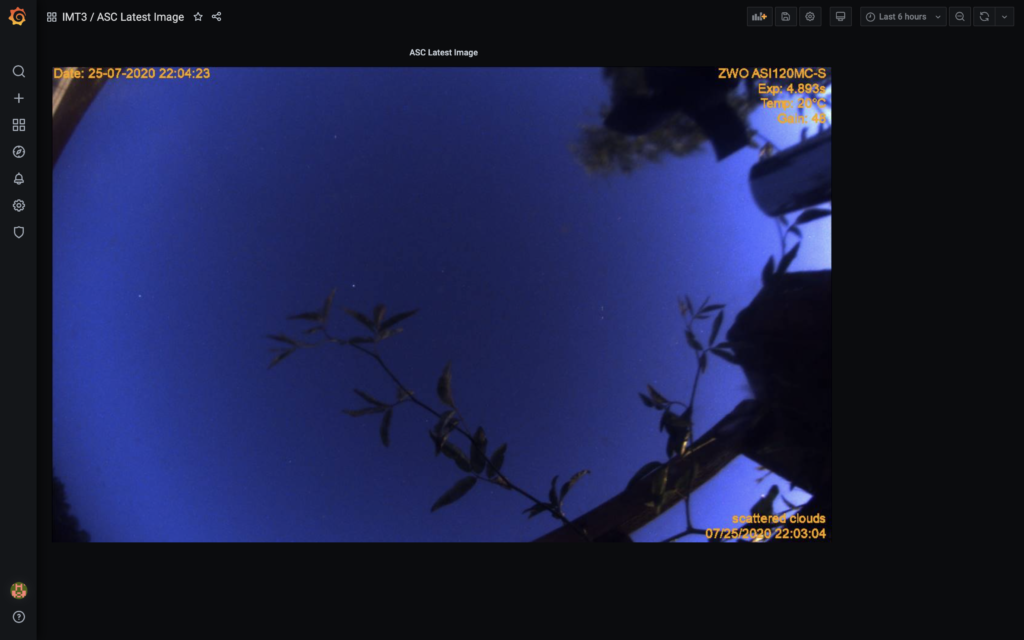

In the image above, we simply add all the subframes from a single filter with the ImageIntegration tool to create the reference image. To create this reference image, we can simply integrate all subframes prior to registering them into a master image using ImageIntegration. The first step in the workflow to properly detect the defective lines is to have a reference image with a noise level as low as possible, in order to detect all of the defective lines that could emerge after composing the master light. For the same reason, they cannot be properly added to a column defect list in CosmeticCorrection, since they are not easily visible by inspecting a single subframe. Most of these lines cannot be rejected by the image integration process since they are very close or under the noise floor of single subframes. In the mouse over below you can see how some of the lower contrast line defects emerge in the higher-SNR master light. SNR increases for the objects in the image, but it also increases for the linear pattern since this pattern remains the same througout the subframe set. This pattern becomes more visible when we register and integrate all subframes into a master light, because this integrated image has a much higher signal to noise ratio. In the following image there is a linear pattern that cannot be completely visible since most of the lines are below the noise floor of the image. The algorithms implemented in these scripts use multiscale and statistical techniques to isolate the lines from large-scale diffuse structures and bright objects in the image that could interfere in the calculation of the properties of the lines to be subtracted. This is done in two main steps: first, we detect the linear pattern to be corrected with LinearDefectDetection, and then we correct the defects in each subframe with LinearPatternSubtraction. If you need to correct rows in your images, you should simply change a parameter in both scripts, as detailed in the next sections.īy using the scripts presented here we'll correct defective columns in individual subframes. LinearDefectDetection and LinearPatternSubtraction can work to correct columns or rows, but in this text we'll be speaking of columns. In the example presented here we'll correct defective columns in subframes. We propose here a methodology to overcome this problem with a new workflow and the use of two new scripts: LinearDefectDetection and LinearPatternSubtraction.

The linear structures are buried in the shot noise of each subframe and only become visible when the images are averaged into a master light. CosmeticCorrection can be applied when you have very few defects in the image, but cannot be applied when your defective columns amount to a 20% for example, because in such case you would be painting a 20% of the image.Īnother reason why CosmeticCorrection cannot be applied to this data set is because the master lights are comprised of up to 120 subframes. The main difference between CosmeticCorrection and CanonBandingReduction is that the first one replaces a defective column with an average value calculated from its neighboring columns, while the latter corrects the banding effect using statistical methods, preserving the original data of defective pixels. Defective columns should be corrected in a way similar to the CanonBandingReduction script. We cannot use a tool like CosmeticCorrection on these images because we would be painting a significant part of the images. A significant portion of the columns in this sensor are now defective, though they still can capture valid data. The scripts presented here were written because of the need of creating master lights from images acquired with a CCD camera having an ageing image sensor. By Vicent Peris (PTeam/OAUV) Published December 23, 2019
